Balancing Risk and Opportunity: Implementing LLMs with a Security-First Approach
Anjali Chaudhary
•7 min read
- LLM training and enhancement
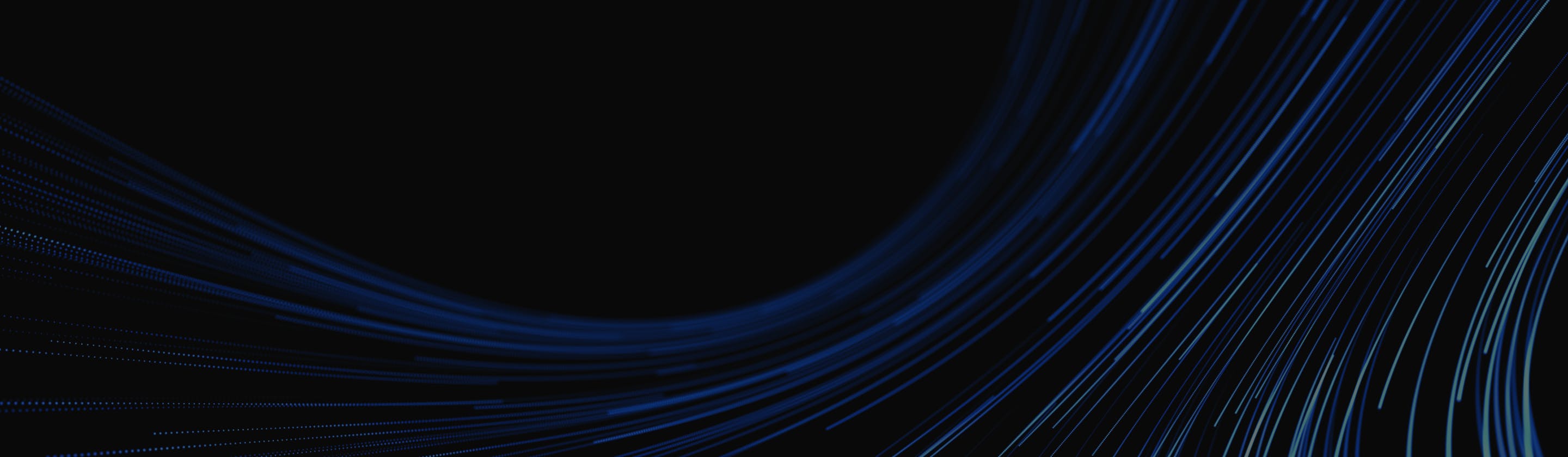
- 1. Prompt injection attacks
- 2. Sensitive information disclosure
- 3. Supply chain vulnerabilities
- 4. Data & model poisoning
- 5. Improper output handling
- 6. Excessive agency (Over-autonomy)
- 7. System prompt leakage
- 8. Vector & embedding weaknesses
- 9. Misinformation & AI hallucinations
- 10. Unbounded resource consumption
- Secure data handling & prevent information leaks
- Implement robust prompt security to prevent injection attacks
- Strengthen API security & third-party integrations
- Prevent model & training data poisoning
- Enforce human oversight in critical AI decisions
- Align AI security with regulatory compliance
- Limit LLM resource consumption & API abuse
Large language models (LLMs) are transforming industries—from enhancing scientific research and legal analysis to virtual assistance and content generation. A McKinsey research states that “Generative AI’s (genAI) impact on productivity could add trillions of dollars in value to the global economy.” However, the rise of LLMs has also introduced new security vulnerabilities that can lead to data leaks, financial fraud, and misinformation.
According to the OWASP Top 10 for LLM Applications 2025 [1] report, key risks include prompt injection, sensitive data leaks, and data & model poisoning, which can result in compliance violations, legal liabilities, and reputational damage.
To mitigate these risks, businesses must adopt a security-first approach—understanding the top threats and implementing AI security best practices for safe, compliant LLM deployment.
Business use cases of LLMs and examples of potential risks
The transformative impact of LLMs is notable in significant business use cases, including:
- Scientific research: LLMs help analyze data and generate hypotheses, speeding up research. But biases in the models can lead to flawed outcomes, skewing results. For instance, a study on AI-generated news content [2] found significant gender and racial biases, which could similarly impact scientific research if not checked.
- Legal document review and analysis: LLMs speed up reviewing legal documents, but they might miss critical or sensitive information due to training data gaps, posing legal risks. A Stanford study [3] showed LLMs often make mistakes in legal tasks, like summarizing cases inaccurately, which could lead to legal errors.
- Copilot assistants: In coding, LLMs assist developers by generating code snippets, boosting productivity. However, they can produce insecure code, threatening software security. Research highlighted that poisoned LLMs [4] can suggest vulnerable code, which developers might use without checking, risking security breaches.
- Content creation: LLMs create marketing materials and content, enhancing efficiency. Yet, they risk producing misinformation, compromising authenticity. The Register [5] reported LLMs create more convincing misinformation than humans, raising concerns about false information spreading.
- Virtual tutoring: LLMs offer personalized educational content, aiding virtual tutoring. The risk is exposing student data, which can breach privacy if not managed. Guidelines from Caddell Prep [6] and the National Student Support Accelerator [7] stress the need for secure data handling to prevent leaks.
- AI-driven chatbots: LLMs power chatbots for efficient customer service, but they may leak personal information or provide incorrect, biased responses. WIRED reported chatbots can guess personal details [8] from chats, posing privacy risks, and the Dutch DPA warned of data breaches from chatbot use [9].
Top 10 LLM security risks
Below are the most pressing LLM security risks:
1. Prompt injection attacks
Attackers manipulate LLM prompts to bypass security controls, inject unauthorized instructions, or force models into unsafe behavior. This can lead to misleading outputs, information leaks, or unintended actions performed by AI-driven applications.
- Example: Cybercriminals might use indirect prompt injection to trick ChatGPT into generating phishing emails.
- Prevention: Filter and sanitize user inputs to prevent malicious prompts, and restrict model permissions to avoid unintended actions.
2. Sensitive information disclosure
LLMs may inadvertently leak confidential, personal, or proprietary information in responses due to improper data handling or model training on sensitive content. If not mitigated, this can lead to privacy violations, legal penalties, and data breaches.
- Example: Samsung employee [10] unintentionally leaked trade secrets via ChatGPT.
- Prevention: Anonymize and encrypt sensitive data before processing, and monitor output logs to detect and block unauthorized disclosures.
3. Supply chain vulnerabilities
Many LLM applications rely on third-party AI models, plugins, or datasets, which can introduce hidden security flaws, backdoors, or malicious components if not properly vetted. Attackers can exploit these weaknesses to inject harmful code, steal data, or compromise model behavior.
- Example: Malicious LLM plugins can exfiltrate data and bypass security filters.
- Prevention: Vet all third-party AI integrations for security compliance, and implement API access controls to limit plugin permissions.
4. Data & model poisoning
Attackers manipulate training data or fine-tuning processes to inject biases, backdoors, or misinformation into models, leading to unreliable AI outputs. This can degrade model performance, introduce ethical risks, or allow attackers to trigger malicious behaviors on demand.
- Example: PoisonGPT [11] attack altered an open-source AI model to spread misinformation.
- Prevention: Use only trusted data sources for LLM training, and regularly audit training datasets for hidden biases or manipulations.
5. Improper output handling
LLM-generated outputs may be improperly validated or sanitized, leading to risks like SQL injection, cross-site scripting (XSS), or privilege escalation in connected applications. This can allow attackers to exploit AI-generated content for unauthorized access.
- Example: AI-generated financial reports may contain miscalculations, misleading investors.
- Prevention: Enforce strict validation of LLM outputs before use in automated workflows, and use content moderation filters to catch potential misinformation.
6. Excessive agency (Over-autonomy)
When LLMs are given excessive decision-making power, they can execute unintended actions, make risky financial or operational decisions, or interact autonomously with external systems in ways that create vulnerabilities.
- Example: An LLM-powered customer service bot might approve unauthorized refunds, causing financial losses.
- Prevention: Limit AI decision-making authority in high-risk environments, and require human review for critical AI-driven actions.
7. System prompt leakage
Attackers can extract hidden system instructions, revealing operational logic, security parameters, or even sensitive credentials embedded in AI prompts. This can lead to unauthorized access or prompt injection attacks.
- Example: An LLM’s internal system prompts may be leaked online, revealing operational guidelines.
- Prevention: Do not embed sensitive data in system prompts, and use separate authentication mechanisms for LLM interactions.
8. Vector & embedding weaknesses
LLMs leveraging vector search (RAG) and embeddings can introduce vulnerabilities where attackers manipulate stored data, inject false retrieval results, or extract sensitive embeddings, leading to misinformation or data leaks.
- Example: Attackers can reverse-engineer LLM embeddings to extract proprietary training data.
- Prevention: Use encryption & access controls for embedding storage, and monitor API requests to detect abnormal vector manipulations.
9. Misinformation & AI hallucinations
LLMs sometimes generate false, misleading, or completely fabricated content, known as hallucinations. This can lead to misinformed decision-making, legal consequences, and reputational damage.
- Example: CNET’s AI-generated finance articles [12] contained major errors, undermining trust.
- Prevention: Use Retrieval-Augmented Generation (RAG) to verify AI-generated content, and require human review for high-stakes AI outputs.
10. Unbounded resource consumption
Attackers can abuse AI APIs, flood LLM models with high-volume requests, or trigger expensive operations, leading to excessive cloud costs or degraded performance.
- Example: Attackers might exploit API weaknesses to drive up compute costs on AI platforms.
- Prevention: Enforce rate limits on LLM queries, and monitor for abnormal query patterns to detect abuse.
One weak point can undo everything you’ve built
Get LLM Safety SupportLLM security best practices for 2025
Securing LLMs requires a multi-layered approach, addressing vulnerabilities from training to deployment and real-world usage. Below are detailed best practices that organizations must implement to mitigate risks.
Secure data handling & prevent information leaks
- Implement data anonymization to prevent the model from memorizing personal data.
- Use differential privacy techniques to introduce controlled noise, reducing data reconstruction risks.
- Monitor outputs for unintended information leakage, especially in customer-facing applications.
Implement robust prompt security to prevent injection attacks
- Restrict LLM input formatting to prevent unauthorized instruction modification.
- Use system message hardening to limit model response variations.
- Deploy automated filtering for adversarial inputs, such as encoded attack prompts.
Strengthen API security & third-party integrations
- Conduct security audits of all third-party integrations to detect vulnerabilities.
- Use API authentication mechanisms, such as OAuth 2.0, to control model access.
- Restrict plugin permissions to prevent unauthorized execution of system commands.
Prevent model & training data poisoning
- Verify and sanitize training datasets before ingestion to prevent manipulation.
- Use cryptographic signing for model updates to ensure authenticity.
- Implement anomaly detection to flag unexpected model behavior.
Enforce human oversight in critical AI decisions
- Require human review for AI-generated legal, financial, or medical content.
- Limit model decision-making authority by enforcing review checkpoints.
- Train employees on LLM risk awareness and responsible AI usage.
Align AI security with regulatory compliance
- Implement explainability measures to ensure LLMs provide transparent decision-making.
- Comply with data protection laws by limiting LLM data retention policies.
- Maintain AI audit logs to track LLM interactions and ensure compliance reporting.
Limit LLM resource consumption & API abuse
- Enforce API rate limiting to prevent excessive queries from draining resources.
- Monitor for abnormal usage patterns, such as bot-driven API abuse.
- Implement cost-aware security policies to restrict unauthorized model consumption.
Wrapping up
As organizations integrate LLMs into critical workflows, they face growing risks, including prompt injection, data leaks, misinformation, and unauthorized access. To mitigate these threats, the focus must shift to responsible deployment. Collaboration between data scientists, cybersecurity experts, and regulators is essential to ensuring ethical AI use, privacy, and security.
Beyond security, AI governance frameworks must evolve to address emerging risks. As LLMs gain autonomy, organizations must balance efficiency with ethical responsibility, ensuring AI remains a driver of innovation rather than a liability.
Turing enables organizations to securely integrate LLMs by providing AI-powered solutions and expert talent to fortify AI-driven applications. Our specialists help businesses build, scale, and safeguard AI models with industry-best security practices.
Talk to an expert today and explore how you can build safe, trustworthy AI solutions!
For further reading and to explore the complete list of references cited in this article, please see our Works Cited document.
Worried about LLM risks? Start with secure training
Security issues like data leaks, prompt injection, and model poisoning often start at the training stage. Turing helps you build safe, aligned models from the ground up.
Secure Your LLMAuthor
Anjali Chaudhary
Anjali is an engineer-turned-writer, editor, and team lead with extensive experience in writing blogs, guest posts, website content, social media content, and more.