Navigating AI Transformation Challenges: A Complete Overview
•6 min read
- AI/ML
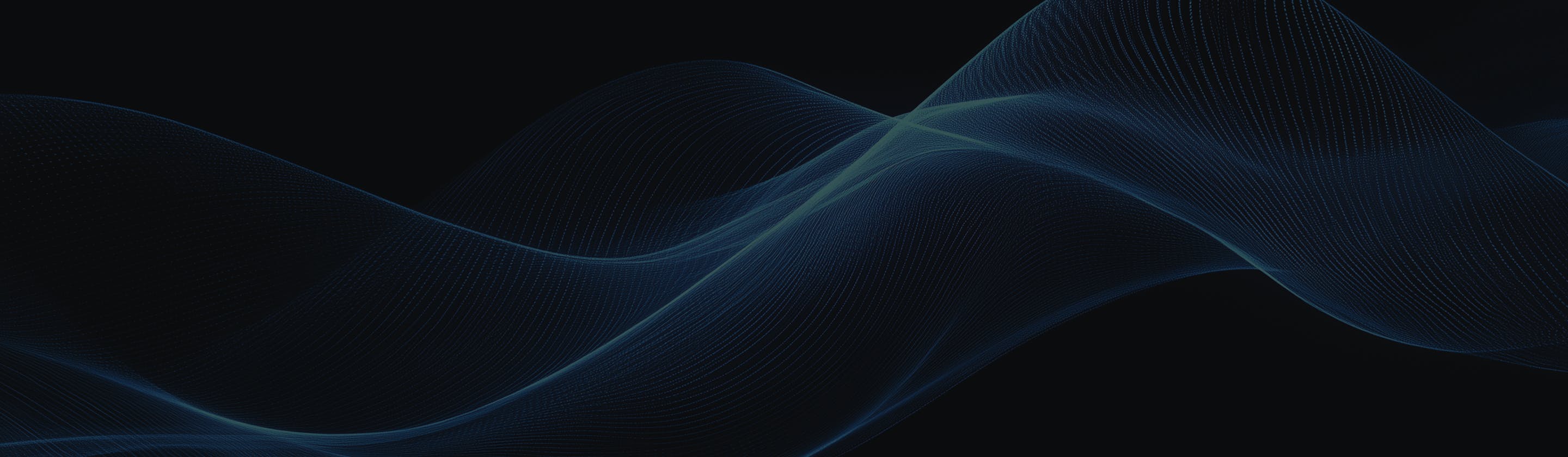
In an era defined by technological advancements, Artificial Intelligence (AI) stands as a significant catalyst for today’s digital transformation. As businesses around the world strive to harness the power of AI, they face a complex landscape replete with incredible opportunities and substantial challenges. To achieve true digital AI transformation, companies must carefully navigate these challenges through a robust AI framework to unleash the power of AI-powered solutions.
At the GDS Data & Analytics Digital Summit 2023, several directors, CIOs, and VPs were surveyed to identify the most significant AI transformation challenges their companies face. These challenges span from data management and a shortage of AI expertise to ethical considerations and the complexities of regulatory compliance. The survey results underscore the need for companies to have a well-defined roadmap to navigate the complexities of AI transformation.
In this blog, we cover multiple AI transformation challenges and offer a path forward to help you build a winning formula in your journey to AI adoption.
Let’s get started!
Navigating prominent AI transformation challenges
There’s an increasing clamor for AI adoption across verticals, however, despite the growing adoption, many organizations still face significant hurdles in their AI transformation journey. According to the survey at GDS Data and Analytics Digital Summit 2023, 35 percent company executives think lack of internal AI expertise is their biggest challenge in AI adoption, whereas 32 percent felt ensuring data quality, privacy, and security was the biggest hurdle.
Quantifying the ROI was the 3rd biggest challenge with 18 percent, while choosing the right models and determining the true cost of AI transformation was voted by 8 percent and 5 percent of participants respectively as their biggest AI transformation challenge. In this section, we document prominent AI transformation challenges and offer a path forward.
1. Lack of internal AI expertise
Although AI has become a buzzword across the tech landscape, there are few organizations that have the relevant AI expertise to properly harness the technology to build sustainable and outcome-driven solutions. AI is a complex field that requires a deep understanding of various technologies, including machine learning, natural language processing, and data analytics. Moreover, implementing AI solutions requires not just technical skills but also the ability to integrate these solutions into existing business processes and workflows.
Recommended approach: To address this challenge, companies must adopt a multi-faceted approach. Investing in AI-training programs and upskilling initiatives can help the current workforce bridge the knowledge gap over the long term. However, having on-demand access to the best global AI talent helps bridge the existing gap, ensuring companies don’t fall behind their competition.
Partnering with expert AI vendors can help you build robust frameworks to mitigate existing challenges and scale based on evolving business needs. These experts assist in identifying the right AI solutions, developing customized models, and ensuring successful implementation. Empowered with the right support, businesses can unlock the full potential of AI to drive strategic transformation.
2. Ensuring data quality, privacy, and security
The foundational success of AI initiatives relies heavily on the quality of data inputs. Suboptimal data pipelines and data protection undermine AI model performance, erode trust, and potentially lead to regulatory issues and data breaches. Simultaneously, the ethical responsibility of safeguarding data privacy and security is paramount, especially in the times characterized by increased data breaches and security risks.
Recommended approach: Companies need robust data governance, security guardrails, and effective logging to ensure resilient end-to-end data infrastructure. Robust data governance practices include establishing data-quality standards, conducting regular data audits, and implementing data-cleansing techniques to ensure the accuracy of data used for AI models.
Following security best practices such as stringent access controls, encryption mechanisms, and anonymization techniques goes a long way in ensuring the privacy and security of your data. Companies must also adhere to privacy regulations such as GDPR to protect customer data and build trust with their stakeholders.
3. Quantifying the ROI in AI
Accurately measuring return on investment (ROI) in AI is tricky, as it mandates tracking costs, benefits, and long-term impact on business in a rapidly evolving field. AI projects often involve multiple components, such as data acquisition, model development, infrastructure, and ongoing maintenance. Estimating the costs associated with these components and aligning them with the expected benefits can be complex. Additionally, traditional ROI metrics may not adequately capture the full value generated by AI initiatives.
Recommended approach: An effective way to quantify the ROI is by following a proven playbook where you build and deploy a low-cost minimum viable product (MVP) for value discovery. Upon successful validation, it can be iteratively scaled to realize outsized business value. This approach ensures reliable investment in strategic AI initiatives as companies can discover tangible business benefits before deciding to allocate large-scale resources to the project.
Besides iterative scaling, companies must establish relevant key performance indicators (KPIs) that align with the organization's objectives to track the progress and impact of AI initiatives more effectively. This data-driven approach can provide valuable insights into the ROI and guide financial decisions that sync with deliverable outputs.
4. Choosing the right models
With multiple proprietary and open-source models in the market choosing the right models for your AI initiatives is a significant challenge that businesses face. The different models have their strengths, characteristics, and challenges, and understanding how these models fit in with your business workflows is crucial. As the AI space continues to evolve rapidly and new models emerge, new layers of complexity are added to the decision-making process.
Recommended approach: To navigate this challenge, businesses must adopt a strategic approach. First, you should clearly define your business use cases and identify the key features you need in an AI model. This approach will help narrow down the options and make the selection process more manageable. Businesses can seek expert AI consultation to understand the nuances of different models.
Next, businesses should experiment with different models on a small scale before making a significant investment. This will allow you to assess the performance of different models in real-world scenarios and guide informed decision-making. It’s worth noting that the objective is not to pick the most powerful model but the one that best fits your business needs.
5. Determining the true cost of transformation
AI transformation holds immense promise, but determining the true cost of this journey remains a significant challenge for organizations. It's not just about the direct expenses involved in acquiring AI tools and technologies; it's about understanding the entire spectrum of costs that includes infrastructure, software, training, operational overhead, ongoing maintenance, and potential expenses as the project evolves.
Recommended approach: Companies must conduct a thorough cost analysis that measures the costs of each AI project component, including hardware, software, data, personnel, and infrastructure. Furthermore, they must assess factors like scalability, maintenance, and potential upgrades to understand the full cost of AI transformation.
To help you with a thorough analysis, enterprises must seek expert AI advisors to fully evaluate multiple workflows and weigh the cost of transformation against the potential upside of your business outcomes. These experts can help organizations identify cost-saving measures, evaluate different implementation options, and provide guidance on budget allocation. A comprehensive cost analysis, consideration of long-term implications, collaboration with experts, and conducting pilot projects provide a clearer understanding of the true cost of AI transformation.
Wrapping up
With the AI landscape evolving rapidly, the lack of internal AI expertise is one of the biggest roadblocks companies face in their journey to successful AI adoption. Besides that, ensuring the quality, privacy, and security of data needed to train/finetune AI models is another significant challenge companies encounter. To navigate these challenges and maximize AI-driven business outcomes, companies must have a well-defined strategy where they identify their business requirements and build a change management plan in collaboration with AI-expert vendors.
At Turing, we have developers from 100+ countries experienced in AI services, cloud, data engineering, full stack, mobile, and 100+ tech stacks, ensuring you get access to high-end expertise for your varied AI projects. Turing has extensive experience in deploying, training, and fine-tuning various AI models and over the last 18 months, we have assisted 50+ clients on various AI projects. This vast experience has enabled us to build robust products and quality control processes ensuring high data quality, and deliver excellent ROI for the clients.
Talk to an expert today!
Want to accelerate your business with AI?
Talk to one of our solutions architects and get a complimentary GenAI advisory session.
Get Started