Inside the Turing Applied AGI Benchmark for VLM 1.0
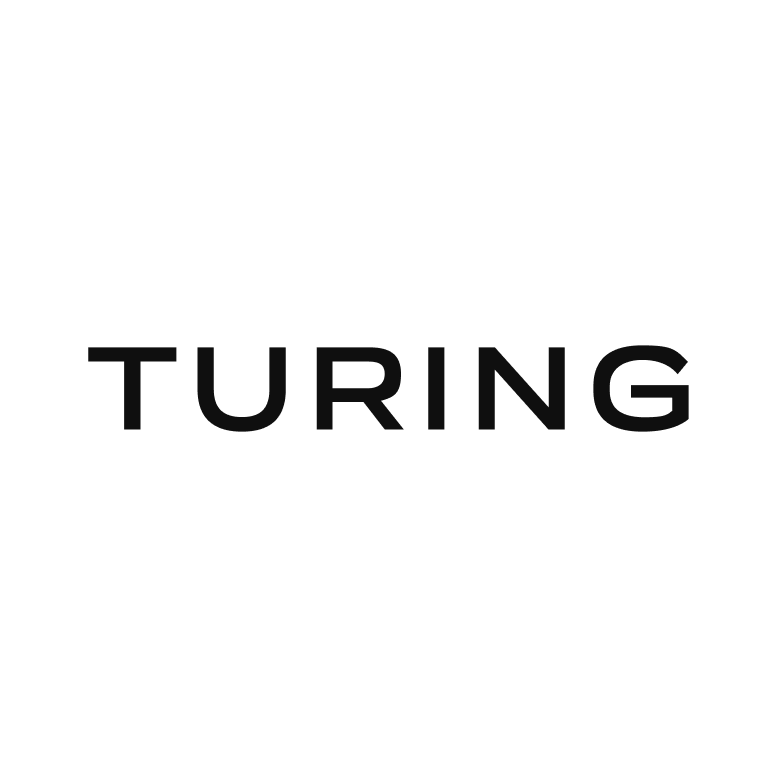
Turing Staff
•3 min read
- LLM training and enhancement
- AI/ML
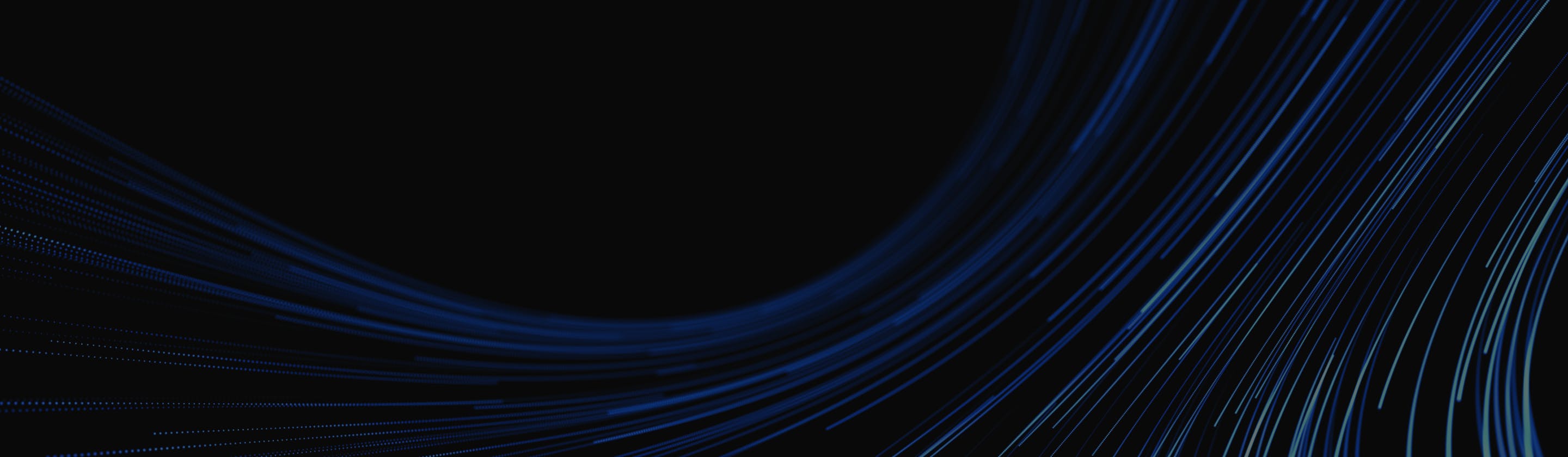
Cutting-edge vision-language models (VLMs) have shown impressive gains on standard benchmarks—but they often fail in the kinds of tasks professionals encounter every day.
Can a model extract figures from a financial report and explain quarterly performance? Can it reason through a schematic and solve a design problem? These aren’t abstract questions—they’re real bottlenecks in applying VLMs in production settings.
The Turing Applied AGI Benchmark for VLM 1.0 was developed to evaluate these challenges directly. Built by Turing’s research team, this benchmark tests VLMs across high-value scenarios in business and STEM domains, combining open-ended text and technical visual inputs.
Below is a preview of what the full technical report covers.
What this benchmark tests
Unlike traditional VQA benchmarks or image-captioning tasks, the Turing VLM benchmark measures performance on open-ended, multimodal prompts that mirror real decision-making environments.
Each task combines text and image inputs and is evaluated by an LLM-as-a-judge scoring system.
The benchmark assesses nine core capabilities:
- Advanced perception – Interpreting dense visuals (e.g., charts, tables, diagrams)
- Spatial reasoning – Understanding configurations and geometric relationships
- Numerical reasoning – Calculating, comparing, and interpreting trends
- Logical inference – Drawing conclusions from complex, multimodal input
- Temporal reasoning – Interpreting sequences and changes over time
- Contextual commonsense – Applying implicit professional knowledge
- Analogical reasoning – Drawing parallels across domains
- Counterfactual reasoning – Responding to “what if” scenarios
- Iterative reasoning – Solving multi-step problems with chained logic
Why it’s different
This benchmark goes beyond recognition tasks or multiple-choice formats. Every task is open-ended and scored on a scale from 0.0–1.0, supporting partial credit and multiple valid answer paths.
The dataset includes:
- ALL subset – Tasks where at least one top-tier VLM fails
- HARD subset – Tasks where average accuracy is below threshold across all models
- A two-level domain taxonomy spanning business, STEM, and technical operations
Each task is validated by domain-expert annotators and undergoes multi-layered review for relevance, realism, and evaluative clarity.
Key early findings
The full report analyzes performance across four top VLMs. Some highlights include:
- Up to 90% degradation in model performance when reasoning and stability are required
- Consistent underperformance on iterative, counterfactual, and domain-specific tasks
- Gemini 2.5 Preview shows leading—but still incomplete—performance on spatial and temporal reasoning
The report also breaks down average scores by capability, offering a clear view of where VLMs succeed—and where they still fall short.
What’s next
Turing plans to expand the benchmark with:
- New domains, including healthcare, legal, and scientific workflows
- More complex visual inputs (e.g., scanned forms, multi-image documents)
- A more fine-grained, rubric-based evaluation protocol
- Opportunities for industry participation and controlled model evaluation access
Get the full report
The complete technical report features capability-by-capability analysis, methodology details, dataset breakdowns, and benchmark results across leading VLMs.
Get Access to the Turing Applied AGI Benchmark for VLM 1.0 Report →
You’ll receive the full PDF as soon as it’s live, along with updates from the Turing Applied AGI Benchmark initiative.
Want to accelerate your business with AI?
Talk to one of our solutions architects and get a complimentary GenAI advisory session.
Get Started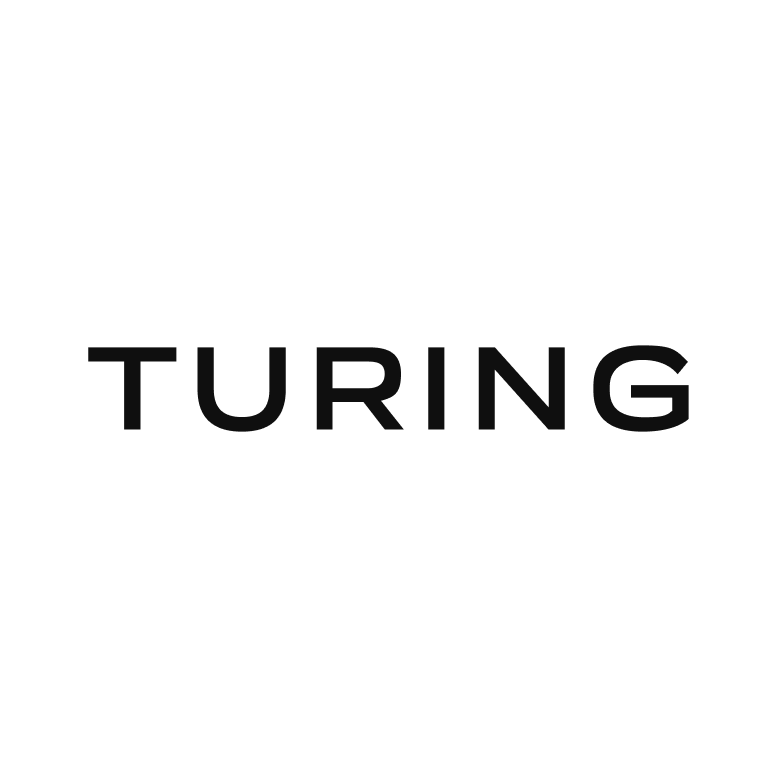
Author
Turing Staff